The National Cancer Advisory Board (NCAB) ad hoc Informatics Working Group (IWG)
Established in mid-2011 by the NCAB, the IWG is charged with providing strategic guidance and direction on NCI informatics investments that support the Institute’s scientific goals. The IWG provides a venue for identifying high-priority biomedical informatics needs, harmonizing ongoing and proposed informatics projects across NCI programs, both intramural and extramural, and reducing redundancies wherever possible. The IWG will explore informatics efforts, potential initiatives, and business models that are in accord with the changes that have occurred in the conduct of cancer research, the practice of oncology, and research administration.
The NCIP Informatics Council
Established in September 2012, the Informatics Council will ensure strategic alignment across NCI award and training programs in biomedical informatics and provide guidance so that NCI CBIIT and its principal program initiative, the NCIP, can prioritize projects and activities. The Council will also serve as a venue for CBIIT and NCIP Working Groups to bring forward strategic recommendations based on interactions with the wider biomedical informatics community, which will further the Institute’s bioinformatics goals in support of scientific research.
NCIP Working Groups (WGs)
CBIIT will convene WGs in focused subject areas. The goals of these groups will be to
- facilitate extramural input into NCIP priorities,
- provide subject-matter expertise in the specific areas around which the groups are convened, and
- support the definition of informatics requirements and priorities in specific domains.
These groups may be established and dissolved over time, as needs dictate, based on the recommendations of the Council. WGs will have representation from one or more appropriate NCI DOCs (Divisions, Offices, and Centers), as well as from key extramural scientific and informatics programs in the cancer research community.
The first three WGs established by the Council are in the areas of High-throughput Molecular Data, Imaging Informatics, and Next-Generation Semantic Infrastructure.
The High-throughput Molecular Data WG will examine NCI program needs and priorities for the management, analysis, aggregation, and integration of data generated by high-throughput technologies; both basic research and clinical applications of these data will be considered. The initial focus will be on informatics initiatives for next-generation sequencing data, given the rapid advances and significant needs pertaining to these rapidly growing data collections. The group’s long-term objectives include
- Lowering barriers to accessing and using data and high-throughput analytical tools
- Encouraging a philosophy of sharing tools, data sets, and expertise
- Providing essential resources for NCI research
- Fostering partnerships to sustain collaborations in the community
The Imaging Informatics WG has the goal of examining NCI program needs and priorities for imaging informatics solutions across the cancer research continuum, from basic and preclinical studies (including small-animal imaging) through translational and clinical research. This continuum also requires standardizing and validating research algorithms and technologies for analyzing enormous amounts of imaging data and metadata in ways needed to satisfy the regulatory requirements set by the U.S. Food and Drug Administration (FDA). The group’s long-term objectives include
- Lowering barriers to performing imaging research with new modalities and analytical tools
- Encouraging a philosophy of sharing tools, data sets, and expertise
- Providing essential resources for NCI (and eventually the larger community)
- Fostering partnerships to sustain collaborations in the community
- Building a sustainable, collaborative community within NCI and subsequently the larger research community
The Next-Generation Semantic Infrastructure WG will identify and prioritize high-level needs as the semantic infrastructure is implemented. As its components are developed, the group will validate the infrastructure through application to a number of selected biomedical research projects. The group will identify projects with specific, unmet needs that can be addressed by the semantic infrastructure, and validate that it is effective in meeting those needs. Where needs are not met by existing semantic infrastructure, the working group will assess the generalizability of the need across multiple projects, and determine whether creating new or evolving existing infrastructure to meet these needs is warranted. The group’s long-term objectives include
- Identifying needs in the NCI research community that the semantic infrastructure can address, with a focus on enabling interoperability and data-sharing that expedite and facilitate projects within and across scientific domains
- Identifying scientific projects where project teams can apply the semantic infrastructure to validate its practicality and applicability
- Lowering barriers to the access and use of data through the provision of semantic infrastructure tools and methodologies
- Encouraging a philosophy of sharing tools, data sets, and expertise
- Providing essential resources to NCI (and eventually the larger community)
- Fostering partnerships to sustain collaborations in the community
Share this Article with others
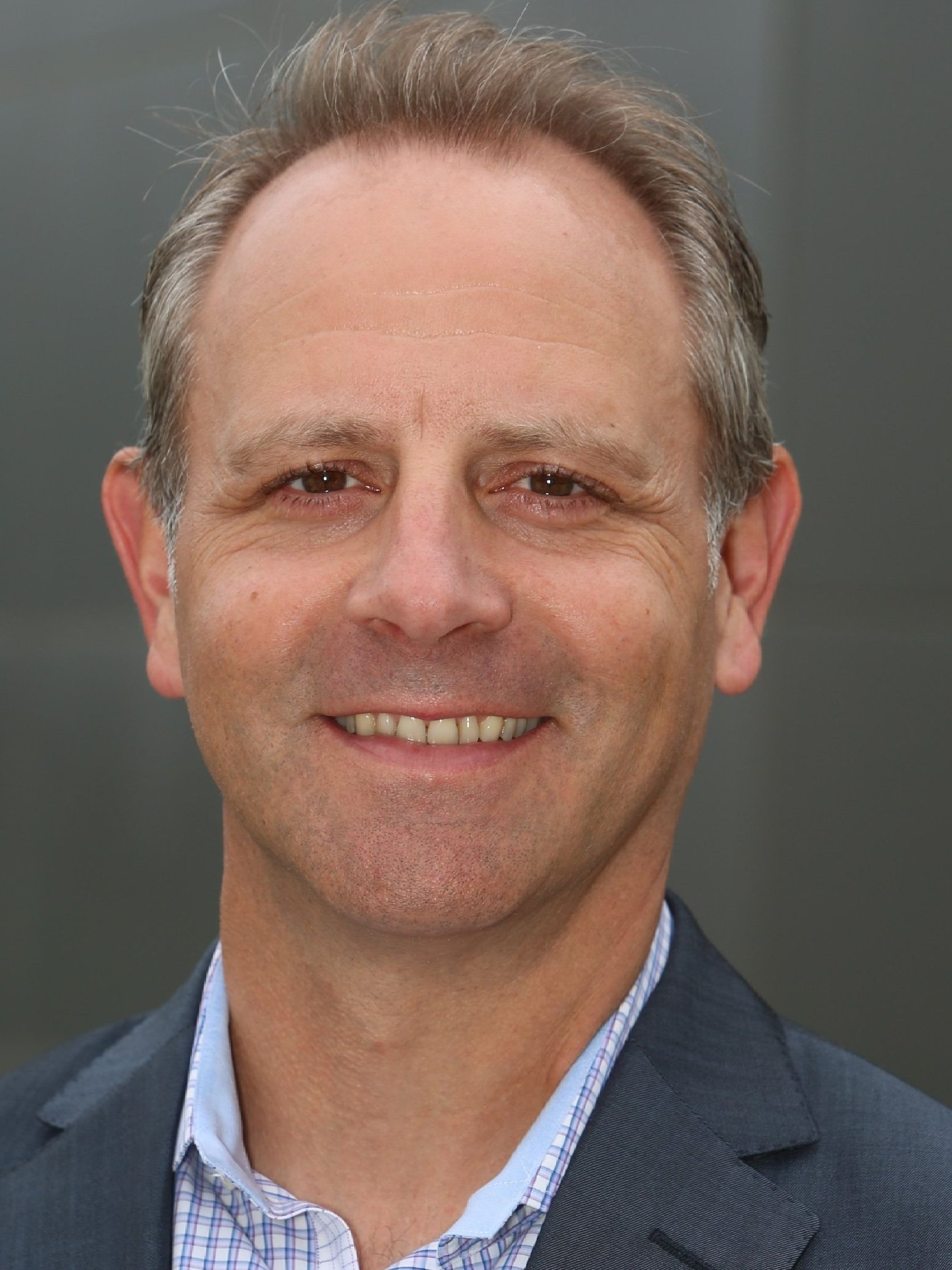